일 | 월 | 화 | 수 | 목 | 금 | 토 |
---|---|---|---|---|---|---|
1 | ||||||
2 | 3 | 4 | 5 | 6 | 7 | 8 |
9 | 10 | 11 | 12 | 13 | 14 | 15 |
16 | 17 | 18 | 19 | 20 | 21 | 22 |
23 | 24 | 25 | 26 | 27 | 28 | 29 |
30 |
- 코딩테스트
- pytorch
- transformer
- Computer Vision
- 딥러닝
- cnn
- Python
- 머신러닝
- 논문
- object detection
- 논문리뷰
- 프로그래머스
- 파이토치
- 파이썬
- 코드구현
- ViT
- 옵티마이저
- 논문구현
- Self-supervised
- opencv
- 알고리즘
- optimizer
- Segmentation
- 논문 리뷰
- Paper Review
- Convolution
- 인공지능
- Semantic Segmentation
- Ai
- programmers
- Today
- Total
목록전체 글 (115)
Attention please
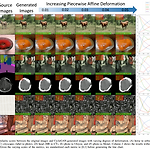
이번에 리뷰할 논문은 SAMScore: A Semantic Structural Similarity Metric for Image Translation Evaluation 입니다. https://paperswithcode.com/paper/samscore-a-semantic-structural-similarity/review/ Papers with Code - Paper tables with annotated results for SAMScore: A Semantic Structural Similarity Metric for Image Translati Paper tables with annotated results for SAMScore: A Semantic Structural Similarity Met..
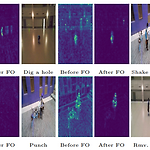
이번에 리뷰할 논문은 FAR: Fourier Aerial Video Recognition 입니다. https://paperswithcode.com/paper/fourier-disentangled-space-time-attention-for Papers with Code - FAR: Fourier Aerial Video Recognition 🏆 SOTA for Action Recognition on UAV Human (Top 1 Accuracy metric) paperswithcode.com 일반적인 image classification 문제의 경우 위 그림과 같이 이미지 내 객체의 class를 분류하는 것을 목표로 하고 있습니다. 이미지 내 객체가 어디에 있는지 위치와 상관없이 종류가 무엇이냐에만 관심이..
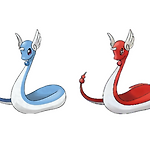
Why Did We Start This Project? 이번 프로젝트는 생성 모델 중 캡션을 기반으로 하여 이미지를 변환시키도록 학습된 instruct pix2pix 모델을 활용하여 채색이 되지 않은 캐릭터 사진을 단순히 텍스트만으로 색을 칠하도록 하는 시스템을 만들어보고자 시작하게 되었습니다. 위 사진과 같이 원래 캐릭터의 모습은 왼쪽 이미지와 같습니다. 전체적으로 푸른색을 띄고있는 캐릭터이죠. 하지만 본 프로젝트에서 개발한 시스템을 기반으로 다음과 같이 텍스트를 입력해주었습니다. Turn it into a creature with a white belly and a red face and back. 간단하게 설명하자면 기존 캐릭터의 푸른색을 띄고 있는 부분을 붉은색으로 색을 칠하라고 캡션을 입력해주었..
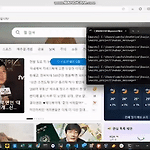
이번에 진행한 프로젝트는 python 패키지 중 매크로를 제작할 때 유용한 pyautogui를 사용하여 간단한 카카오톡 예약 메세지 기능을 구현해보았습니다. 사실 카카오톡 자체에는 예약 메세지 기능이 존재합니다. 해당 기능은 1:1 톡방이나 단톡방에서는 사용이 가능하나 오픈채팅방에서는 예약 메세지 기능이 존재하지 않습니다. 물론 오픈채팅방에 예약 메세지를 보낼 일이 별로 없어서 불편함을 느끼진 않았지만 이번에 학교에서 진행하는 데이터 청년 캠퍼스 에서 조교를 맡다보니 출석 메세지를 10시, 10시30분, 1시30분, 2시에 보내야하는 문제가 생겼습니다. 물론 수동으로 메세지를 보내도 되긴 하지만......요즘 방학 시즌이다보니 밤낮이 바뀌는 바람에 오전에 일어나기가 너무 힘들더라구요..ㅠ 그래서 이번 기..
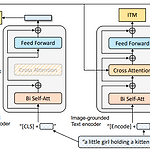
이번에 리뷰할 논문은 BLIP: Bootstrapping Language-Image Pre-training for Unified Vision-Language Understanding and Generation 입니다. https://paperswithcode.com/paper/blip-bootstrapping-language-image-pre Papers with Code - BLIP: Bootstrapping Language-Image Pre-training for Unified Vision-Language Understanding and Generation #3 best model for Open Vocabulary Attribute Detection on OVAD-Box benchmark (mean..
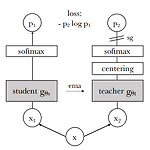
이번에 리뷰할 논문은 Emerging Properties in Self-Supervised Vision Transformers 입니다. https://paperswithcode.com/paper/emerging-properties-in-self-supervised-vision Papers with Code - Emerging Properties in Self-Supervised Vision Transformers #2 best model for Visual Place Recognition on Laurel Caverns (Recall@1 metric) paperswithcode.com Introduction ViT(Vision Transformer) 는 최근 CV(Computer Vision) 분야에서 ..